Le CRIL en bref
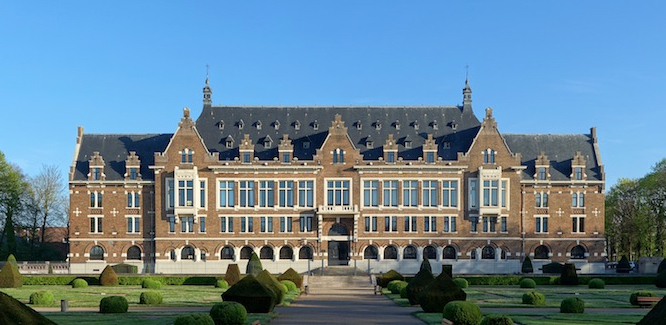
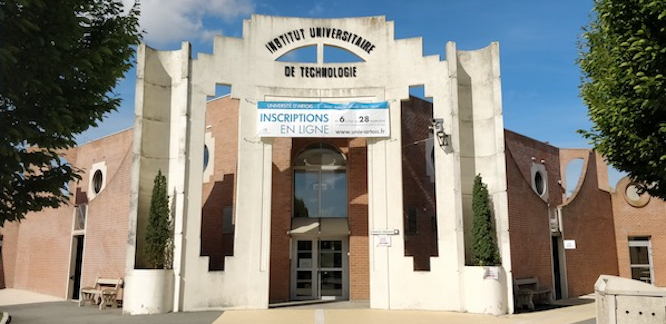
Le Centre de Recherche en Informatique de Lens (CRIL UMR 8188) est un laboratoire de l’Université d’Artois et du CNRS dont la thématique de recherche fédératrice concerne l'intelligence artificielle et ses applications. Il regroupe près de 70 membres : chercheurs, enseignants-chercheurs, doctorants et personnels administratifs et techniques.
Le CRIL participe à la Confédération Européenne de Laboratoires en Intelligence Artificielle CLAIRE et à l'alliance régionale humAIn. Il bénéficie du soutien du Ministère de l’Enseignement Supérieur et de la Recherche, du CNRS, de l’Université d’Artois et de la région Hauts de France.
Le CRIL est localisé sur deux sites à Lens : la faculté des sciences Jean Perrin et l’IUT.
Publications récemment mises à jour
Articles de conférences internationales
Actualités (RSS)
Séminaire Séminaire de Arthur Ledaguenel - IRT SystemX
Neural Classification Informed by Prior Knowledge
20 juin 2024 - 14:00Neurosymbolic artificial intelligence is a growing field of research aiming to combine neural networks learning capabilities with the reasoning abilities of symbolic systems. We introduce a formalism for supervised multi-label classification informed by prior knowledge. We build upon this formalism to re-frame three abstract neurosymbolic techniques based on probabilistic reasoning. We then evaluate experimentally and compare the benefits of all three techniques across model scales on several informed classification tasks. Finally, we discuss the computational complexity of probabilistic reasoning, which is of cardinal importance to assess the scalability of probabilistic neurosymbolic techniques.
Séminaire Séminaire de Guillaume Lagarde : Université de Bordeaux, LaBRI
Scaling Neural Program Synthesis with Distribution-based Search
4 avr. 2024 - 14:00In this talk, we will discuss the problem of automatically constructing computer programs from input-output examples, especially when the target language is domain-specific and defined using a context-free grammar. I will introduce a theoretical framework called distribution-based search, discuss its challenges, and present several search strategies based on learning the weights of a probabilistic context-free grammar (PCFG) and then using this PCFG to enumerate the most promising candidate programs efficiently. The presentation will be based on the following paper published at AAAI'2022: https://arxiv.
Séminaire Séminaire de Vincent Derkinderen KU Leuven
ProbLog: Harnessing Knowledge Compilation for Probabilistic Inference in AI
29 févr. 2024 - 14:00Probabilistic reasoning is a key aspect of artificial intelligence. In this talk we will look at probabilistic reasoning through the lens of ProbLog, a probabilistic logic programming language. This language extends the logical facts and rules of Prolog with probabilistic facts. Probabilistic reasoning in this language is achieved through knowledge compilation techniques. We will delve deeper into the details of this reasoning pipeline and highlight some of ProbLog's extensions. In particular we consider DeepProbLog, which heavily benefits from inference through d-DNNF compilation.
Séminaire Séminaire Emmanuel Lonca, CRIL.
Clustercril v2024: CRIL cluster, Slurm & good practices
22 févr. 2024 - 14:00The CRIL cluster has been providing the laboratory's researchers with computing resources since it was set up in 2009. Since then, the evolution of these resources has been continuous, leading to a heterogeneous architecture in which traditional computing nodes, nodes dedicated to distributed computing, GPU nodes and various servers to drive this computing power cohabit, all driven by the Slurm resource manager. The aim of this talk is to present the calculation tools available, and how to use them (correctly) via Slurm.